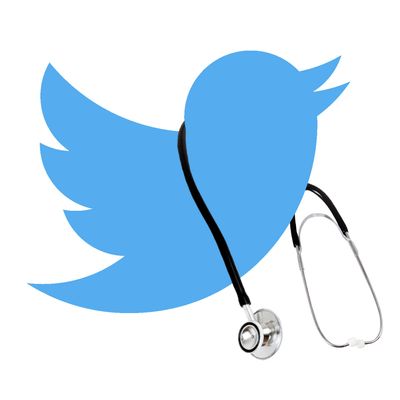
Never before in human history have so many people expressed their emotions so publicly. Every day, countless gigabytes of happiness and sadness and frustration and every other conceivable feeling are dumped onto the web, whether in the form of ecstatic Facebook statuses or paranoid blog posts. Gifted with a mother lode of new psychological data, researchers are eagerly lapping up as much of it as possible in an attempt to better understand homo sapiens.
Naturally, Twitter is a major nexus for this sort of research. And for a new study in Psychological Science, a large team of researchers led by Johannes Eichstaedt, a psychologist at the University of Pennsylvania, found that the sentiments expressed on Twitter were surprisingly effective as a public-health diagnostic device: They could predict, more accurately than a slew of “traditional” health and demographic variables, how frequently people died from atherosclerotic heart disease (AHD) in a given county.
The researchers were intrigued by Twitter’s potential on this front because of past research, which has shown that while behaviors like smoking and drinking increase one’s risk of this form of heart disease, there’s also a psychological component: Depression and stress are risk factors, while “positive psychological characteristics, such as optimism … and social support” can protect against it. In theory, then, places with lots of angry or frustrated people might have higher rates of heart diseases than places populated by Zen masters (all else being equal).
So, the researchers figured, why not examine tweets at the county level, measure the frequency of positive and negative emotion, and see if this can predict risk of death from heart disease? Armed with a big trove of data from Twitter — “148 million county-mapped tweets across 1,347 counties” — as well as mortality statistics from the Centers for Disease Control, they got to work.
Data in hand, they analyzed the sentiment of the tweets (a trickier-than-it-sounds process that they run down in the paper), created a bunch of models attempting to correlate these sentiments and other demographic and health data with mortality levels, and tested which of these models most accurately predicted the prevalence of AHD mortality in a given county. As it turned out, a model based only on tweet sentiment performed slightly but significantly better than a model using all the classic predictors like age, race, and smoking and drinking behavior instead of tweets. (The very best model used both the classic predictors and Twitter, but it didn’t beat the Twitter-only model by a statistically significant margin.)
This is quite a finding, when you think about it: It suggests that all the demographic and health data medical researchers spend a painstaking amount of time gathering together was less useful for predicting AHD mortality in a given county than simply scraping a bunch of tweets from that county. (Which isn’t to imply that that data isn’t still extremely important, of course.)
Overall, while both positive and negative tweets helped the researchers predict AHD death rates, the negative stuff told the researchers more. “We do see more topics predictive of higher mortality rates than those predictive of lower rates,” said Andrew Schwartz, one of the co-authors, in an email, “and we see higher predictive strength for those predicting higher rates of mortality.” Why might this negative stuff have a bigger relative impact? “With this kind of heart disease there should be a direct physiological link between negative experiences and artery health,” Eichstaedt explained in an email. “Positive experiences, on the other hand, don’t have a physiological link to cleaning up your arteries (they down-regulate negative emotion — that’s it). So this kind of heart disease might be more sensitive to negative psychological experiences than positive ones.”
Now, as Eichstaedt and his colleagues note in the paper, tweets might constitute a somewhat biased source of data. For one thing, people cultivate a particular version of their online selves — it’s not as though every emotion they are actually feeling finds its way into a tweet. Moreover, Twitter users are younger and more urban than the population at large. Overall, though, Schwartz said that Twitter users are pretty average in most other ways and therefore good fodder for this sort of study. “We haven’t seen any strong evidence that Twitter users have different psychological characteristics beyond what is explained by demographic and socioeconomic biases,” he explained. “For example, younger individuals are more likely to use high arousal emotional language, but nothing that distinguishes that isn’t explained by demographics or socioeconomics.”
Plus, as far as the researchers are concerned, the proof is in the pudding: Whatever qualms one might have about analyzing Twitter for this purpose, they write, it “captures as much unbiased [heart-disease-]-relevant information about the general population as do traditional, representatively assessed predictors.” Twitter is clearly telling the researchers — telling us — something important about a given area’s population.
There’s one mysterious aspect to all of this. As the researchers note, since the median Twitter user is 31 years old and people at significant risk for AHD tend to be much older, “it is not obvious why Twitter language should track heart-disease mortality. The people tweeting are not the people dying.” Why, then, are their tweets apparently giving us useful information about their communities?
Because, to put it simply, communities affect individuals:
Local communities create physical and social environments that influence the behaviors, stress experiences, and health of their residents… Epidemiological studies have found that the aggregated characteristics of communities, such as social cohesion and social capital, account for a significant portion of variation in health outcomes, independently of individual-level characteristics[], such that the combined psychological character of the community is more informative for predicting risk than are the self-reports of any one individual.
By examining the sentiments of a 30-year-old of Twitter, you’re not just learning about her — you’re learning about her community, including its older, more vulnerable residents. “The language of Twitter,” the authors write, “may be a window into the aggregated and powerful effects of the community context.” It’s no wonder, then, that researchers are so excited about the age of emotional public data.